WWT's Journey into Generative AI: Digital Assistants
AI chatbots are on the rise as businesses aim to meet customer needs and boost loyalty with personalized experiences. However, creating AI assistants is tough due to the wide variety of tools and methods available.
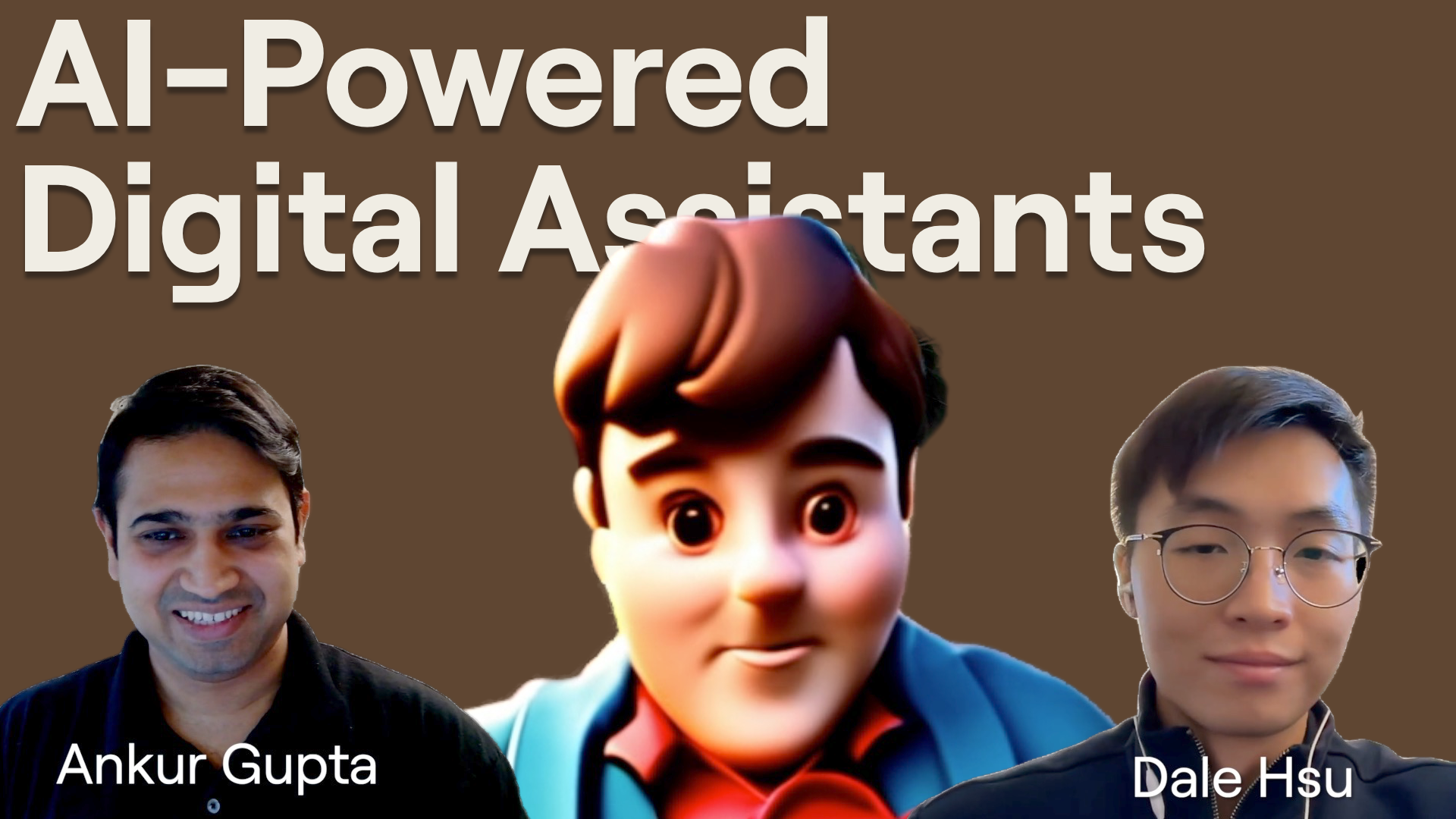
In the last year, we've all been strapped in for the exhilarating ride through the landscape of AI tools. It's been a journey filled with anticipation and discovery, but what truly tests the mettle of these tools is their application within the bustling ecosystem of a successful business. Here, the game changes; the stakes are significantly higher, and the challenges, more complex.
Artificial Intelligence
Artificial Intelligence is hardly the new kid on the block. Its roots have burrowed deep and spread wide over the years, evolving into a force to be reckoned with. Parallel to this evolution, World Wide Technology (WWT) has been honing its expertise in the AI arena for over a decade. This dedication has not gone unnoticed, drawing attention from industry leaders who are keen to understand how to best navigate the burgeoning AI landscape. WWT stands at the forefront, equipped with answers yet also engaging head-on with the challenges that come with being a global powerhouse. With a workforce thousands strong and competition at every turn, the pressure is immense.
Now, three decades into their journey, WWT's insights into AI are as precious as they are rare. It is with great excitement that we bring into the spotlight Ankur Gupta, WWT's esteemed Director of Data Science, hailing from the vibrant tech hub of the Northwest. Alongside him, we have Dale Hsu, a consultant whose expertise has been pivotal in overseeing WWT's internal AI deployments. Dale's role is critical, highlighted by his regular strategy sessions with WWT's CEO, Jim Cavanaugh.
For today's show, we delve into the heart of WWT's innovative venture into generative AI, as detailed in their latest research publication, "How WWT is Harnessing Generative AI to Drive Internal Business Value." Join us as we explore two groundbreaking generative AI projects that WWT has implemented internally. We'll uncover the selection process, the aspirations behind these initiatives, and the tangible outcomes they've achieved. This journey into WWT's AI-driven innovation is not just about technology; it's about pioneering new paths and setting benchmarks in the business world. Welcome to the future, as envisioned and actualized by WWT.
Thank you for watching!
Robb
explainerds.net
Lightly edited transcript below (TLDR)
WWT-2401 Digital Assistants
Recorded Jan 8, 2024, ExplaiNerds.net
Robb Boyd: Over the past year, diving into the world of AI tools has been an exhilarating journey for all of us. But what happens when these tools enter the dynamic world of a thriving business where there's much more at stake? That's where the real challenge begins. AI isn't a newcomer. It's been gaining steadily over the years, and in parallel, World Wide Technology has spent over a decade refining their mastery in this area. It's no wonder that leaders from business and tech sectors are turning to them, asking, "How do we navigate this new terrain?" WWT has answers, yet they're also in the thick of addressing these same challenges. A global giant with thousands of employees and fierce competition, the stakes couldn't be higher.
And with three decades in, their insights are gold, rare and valuable. And joining us today from the caffeinated tech hub of the Northwest, Ankur Gupta, WWT's Director of Data Science, and from the epicenter of cultural heritage and artistic expression, consultant Dale Hsu. Dale plays a crucial role in overseeing WWT's internal AI deployments, a responsibility underscored by his weekly meetings with CEO, Jim Cavanaugh.
Today's show is based on the published research titled "How WWT is Harnessing Generative AI to Drive Internal Business Value." We take a deep dive into two generative AI projects WWT has rolled out for internal use. We'll check out how they picked them, what they hoped for, and the results that they're seeing. I'm your host, Robb Boyd. I firmly believe that the wisdom and triumphs of our predecessors form the bedrock of today's progress. Welcome to World Wide Technology Presents Research, with insights powered by the Advanced Technology Center. Tell us what you do for World Wide and how long you've been doing it.
Ankur: I'm Ankur Gupta, I've been with World Wide for about eight years. I am a data science director here with World Wide, but primarily my background has been in analytics and data science, way before it was called the sexiest job of the century, I think it was, Data Scientist.
Robb Boyd: I missed that one. What are you reading?
Ankur: Back then we were called Big Data.
Robb Boyd: What are you reading that finds out, that calls your profession I like that though. I agree. Come on. Sexiest? That's cool. How often do we ever get that kind of thing?
Ankur: Yeah. At one time it was called the sexiest job of the century and I think it got recently dethroned by Prompt Engineering as being the sexiest job of the century. But yeah, that's what I've been doing for the last 14 years or so. but more, more recently with the World Wide, leading their data science practice, making sure our deliveries to customers in the AI domain are as smooth as possible.
Robb Boyd: Okay. Dale, what is it you do for World Wide? And, how are you going to help us today?
Dale: Hey everyone. My name is Dale. I'm currently a consultant with World Wide Technology. I've been with the company for around three years. in the latest initiative, I help lead our internal Gen AI. Center of Excellence under Ankur so yeah, happy to share everything about Generative AI today.
Robb Boyd: That's perfect because that's exactly where I want to go in this conversation, which is to talk about, we're building this on a research paper that both of you contributed, not only to the research but to the writing as I loosely understand it. But the idea is that you guys are doing things internally with Generative AI that other companies are wanting to do for themselves because it's not been easy to figure out. The hype is so high and there's so many interesting things we can do as individuals. But the smart customers are not wading into this super fast, and throwing money at it, even though it can feel like you might need to do something like that, because things are are operating so quickly and changing so quickly. And so I love where you guys can both speak to us as an enterprise who's doing this for your own users, but then you're plowing the same education and that, and then sharing it with clients, as I understand it. So we're going to talk more about the specific projects that were featured in this research paper, but let's get a good grounding in how far you guys have come with AI.
In general, so Ankur I guess I'll start with you and talk about W. T. S. Vision for A. I believe practical A. I. Is something I've heard you guys say a lot. Do you mind explaining what that is and how it fits?
Ankur: Yeah, even before I get into Practical AI I like WWT . Has been doing A. I. For the last 10 years, by the way, we've had an A. I. Practice and a team for the last 10 years and we've delivered for our customers from like industrial mining up to like financial services and retail. Most recently, the buzz with the AI, we've just reinforced on our messaging that has been the same for the last 10 years, which is Practical AI. Which is, the industry is going to come up with a whole lot of new innovations. Some of those might be the best fit for you. Some may require a bit of tweaking. And sometimes customers just need something totally brand new. So Practical AI is that balance between where you need to build something new or buy something off the shelf. Or buy something off the shelf and kind of build and do a hybrid in between. So that is where we, recommend to our customers, don't go in with, with a set template of I just want to buy, or I just want to build. Let us figure it out for you and recommend to you what would be the right thing there. If it's something as innovative as GenAI I has been for the last one year maybe it's a hybrid. You don't need to build a new LLM, but you can build off of LLMs that Microsoft and the Meta's of the world have built and. just tweak your solution on top of it. That's very similar to what we've been doing internally as well.
Robb Boyd: What are the decisions that need to be understood? Or what are the big things that jump out at you from clients saying, I'm trying to make a build versus buy decision? How should I be looking at this in general, broad strokes across industries?
Ankur: One of the first things we saw with customers was. GenAI specifically, when it came on board, it was like this, holy grail, like this, magic black box where you could just dump in all your data and would have answers. we have to pass some wits over here, which is that you cannot just dump in all your data into an LLM. There is still data governance still is the meat of everything. You still have to have your data in a certain format. You have to have that data accessible. All the silos in your organization still have to be broken down. So all of that still remains. Those foundations are what we build on top of. But essentially, yes, after that, it's all about making sure the LLMs are hooked up right and they can read from your data. We do the indexing, right? We do the chunking, right? We do the, similarity matches, right? And through that effort. We've perfected that art over the last six months or so.
Robb Boyd: And I think that's a great point because I think we tend to, that combined with the fact that when you want to roll your own from a, from a data center perspective, there's plenty of open source projects that I can download and run on a machine. But to think that would scale to an organization or the data that an organization needs to process. is infinitely bigger as I understand it from a machine perspective and the number of GPUs and the compute power. And that's why we see these big extremes of, large companies that are, well versed in the market, able to invest appropriately to be able to get answers like this, but we've got to figure out, what's that balancing act. And this is what you guys sounds have been working on quite a bit.
Ankur: Exactly. And that goes back to practical AI. We see Google and we see Amazon and all the cloud providers coming up with their, products. Which is like, Oh, throw your data in there and you have a jackpot. that works. I'm not saying that doesn't work, but does it really solve the problem that you're trying to tackle with your use cases? Does it actually answer the question that you're trying to get out of those documents? Maybe, not. And that's where we come in with Practical AI. help with all the nitty gritties and proof gains that has taken us the last six months to build something internally within WWT
Robb Boyd: You guys, I read about a kind of a three layer model, about advising where you're spending your time now and why it's important to understand these layers. And I believe this is what you're using this and how you describe to customers about your approach. Can you tell me what is, what is that, what are these three layers and how do they relate to each other and why is that important to understand?
Ankur: What we've observed is that this whole more in context learning or otherwise called RAGS retrieval augmented generation. That's the one picking up, which can get you to results or outcomes really, fast. The main three layers in there are number one, the generative layer, which is basically where your creativity lies and where your synthesis lies. And those are essentially the LLM's that could be an open source LLM, that could be a proprietary LLM from an Amazon or a Meta or a Google or a Microsoft. We don't care about that.
Robb Boyd: Could it be both? Yeah. Could it be multiple? Oh,
Ankur: it could be multiple, yes. It could be a hybrid,
Robb Boyd: yeah. Okay, but the idea is to distinguish it at its own layer.
Ankur: Exactly. But essentially for us, it's a plug and play. there's some minor parameters in there that you could tweak with like temperature settings and stuff like that. But for us, it's plug and play. the meat of the development that needs to be done to get to an outcome are the two layers that we call the context layer and the orchestration or the exchange layer. The context layer is what we were talking about earlier, where you take your proprietary data, your organization data, and do the embeddings on it, do the jolking on it, and basically translate it into a format that is fit for your purpose that you're trying to build in terms of a chatbot. So think about all the metadata in the world that is associated to a file. Who's the author? What's the title? What department does this person belong to? All of that needs to be taken care of in the context layer. And then you go to the exchange layer, which is basically just the bridge between the context layer, the generative layer, as well as the user experience. And it's trying to do all the orchestration around all these things, make sure the caching is done right, make sure the results are timely. So this is also the layer that we see, or we project that a lot of innovation will happen essentially because most of the effort has been concentrated towards the context layer and the generative layer for the last year or so that is where all the people are like investing their time, money and intellect in as these products go more mainstream in terms of like more enterprise grade usage. The exchange layer is found, get all that attention now so that response sites can be quicker, our back can be, put in place and all those good things that come with enterprise applications.
Robb Boyd: But it also, that exchange layer also helps you define how you're going to interact with those modules and keep those things distinct, right? So that you don't, once you've chosen the appropriate. LLM balances based on whatever they are toward what you want to achieve. You forget about those and you focus all your time on the contextual layer, to interact with those things and pull that focuses your efforts, your time and your attention on the results that you want to achieve.
Ankur: No, very good example, over there on what the exchange layer allows us to do. As you said, multiple LLMs, Google's LLM and, Microsoft or OpenAI's LLM, both can be sitting in the generative layer. And based on the complexity of the query coming in, the exchange layer can decide, Oh, the Google API is 50 percent cheaper than the Microsoft one. The Microsoft one is more expensive, but it can handle different queries. So how should I route these queries? Those things can happen in real time and be optimized for cost as well as quality.
Robb Boyd: What I like too is those things improve because everybody's in this arms race to improve with new and better models and very specific models for different things, whether it be visuals or text or moving images or, what is it? vision, computer vision. then those can change and update yet. It won't the way if you design correctly, the way you're describing it, then I assume then all your work on the contextual layer can stay the same and benefit from the updated LLMs. And therefore you can scale more efficiently, uniquely to what you've developed.
Ankur: Except when, multimodal goes mainstream. multimodal will, will have like images and videos and whatnot. And right now I don't even know what the context layer for those might look like. I can only guess. We've started playing around with it. But yes, the concept is that hopefully we shouldn't have to change the context layer. But what if the data changes? There is no text anymore, it's all images and videos. What does that look like then?
Robb Boyd: But what I love about what you just said is that, this is what I like about working with engineers and technical types, is that Are you now acknowledging that things may change? Are you saying that we don't have it all figured out? Of course not. Yeah.
Ankur: That's always the case. Like things change, like change is the only constant, right? So we may still call them the context layer, exchange layer, generative layer, six months down the line. the inner workings might be very different than what they are right now.
Robb Boyd: I want to ask Dale about these internal projects that you guys have been on. These are the features of the paper, but to set this up, I'm gonna stick with you for one minute longer Anker, helping understand how the ATC has changed. and because, this composable AI lab, I don't know if I'm saying that correctly, but you guys have specifically invested in the ATC. first describe what the ATC is and then describe how the, composable labs are starting to, change things there.
Ankur: Yeah, the ATC has really been a differentiator for us at World Wide and our data scientists and our team, the ATC, the main vision or the main objective over there was to allow our customers to get access to the latest and greatest equipment that they essentially want to decide on, like whether they should get company A's version B model or company B's or Z's, something else. So how do you compare that's the ATC, everything that's out there, the latest and greatest into one data center, let people play around and test it out and do combinations and figure out what's the right stack for them. And then take the decision to buy it. Having after, having tried it and as a data scientist, as getting access to all that gear upfront is, it's phenomenal. I remember even two years ago, we used to have access to the best GPUs back then. And, my, my peers or my friends outside of WPT, they were like, Oh, how do you even get access to that kind of stuff? you don't get access to it. It's not for us to spin it up. So it was, fun. and even right now it's taken up so much. for example, NVIDIA's H100s, A100s, these are in such short supply. There's like a one year wait time for our customers to get hands on. And we have those in the a PC right now. So the AI composable lab that we're talking about right now, that's the latest and greatest focus on ai. So the greatest GPUs right now, the, best storage, infrastructure that support supports AI workloads and also like network and all of that stuff. it's getting access to all of that. So it enables us to learn about all that stuff and advise our customers and our customers to come, be hands-on with all of that stuff before they order it.
Robb Boyd: Let me try something on you that I just thought of. ATC is really about moving from theory to practicality. So as you work with someone to say, this is how it should work. These are the components we think based on this, but then it becomes, let's plug it in and make sure that it does what we think it'll do because, 99 percent of the time, there's going to be some changes with that, or you learn something about that vendor's implementation with that model perhaps has these unexpected effects. And that's the kind of stuff we want to find in the lab versus in deployment work. It's super expensive and harder to turn around, right?
Ankur: It also helps us, figure out vaporware from reality. So if there's like a product vendor or someone who's Oh, test my AI product or solution and, see how good it works, we'll be like, Oh, let's, get it up and running at the ATC
Robb Boyd: oh, you have a lab. Yeah, exactly. Oh no. let's go into these two projects that you guys have, embarked upon. And the reason why I love these examples from this paper is that. It's easy to forget that most of us relate to WWT as a vendor. You provide services, you have smart people that I can hire and engage with, but you're also a company and you've got all the same company issues. Everyone else does from regulation to where to make smart investments for the future to, just. being a good, steward of your, of your, stakeholders, so to speak, even though you guys are privately held, but still you guys are very well run.
And first, Dale, let me ask you, cause I think you'd be familiar with this. I assume that the two that we're going to talk about, the ATC assistant, as I know it from the paper, as well as the RFP assistant, these things are two that you started working on, but there was many more being suggested by business units. When asked to say, Hey, what should we work on? And you guys had to go through a criteria of choosing what to focus on. Assuming you're familiar with that. Do you mind explaining what that thought process is and broad strokes and how you settled on these two?
Dale: Yeah, sure. RoRobb, thk the whole process, it's a very lengthy one in this one that came as exactly as ordered from Jim, right? Our CEO. So from the top down, the direction was that, Hey. See guys, Gen AI is a thing that, has sprung into action in the last half year. Could we do something about it internally? if so, where do we start? So we started with the, guidance of going into different lines of businesses to really decide, what do we, what, should we work on first? and I think the first step is for our team, me personally to speak with, a bunch of managers. Up to the VP level, across different verticals with the WVT, such as finance, sales, operation, marketing, to really uncover their use cases. And to go into these meetings, we actually prepare a set of criterias, as you mentioned, from value and complexity across different levers. Within the value piece, there are different levers that we pull, such as what is the use case bottom line impact to WWT in terms of financial value that it brings, operational value that it brings, and also marketing value that it could achieve. On the complexity side, we have help from our data science team to really dive into use case. And this goes back into some of the build versus buy and data governance section that we have discussed earlier on. for each use case, we evaluate the data sources that's associated with it. What is the feasibility of actually bringing the data into the pipeline? And is all the data available to our data scientists for use? So these are the criteria that we went into these meetings evaluating with stakeholders at a very detailed level to really uncover the value and complexity of these use cases before deciding what are the top use cases we should focus on.
Ankur: Yes, on paper, we did it value and complexity, but I think a third dimension we always had in mind was learnability and kind of showmanship and creative nuance and stuff like that, because this was all new. Everyone had to learn from scratch on here. So it was like, Oh, which one of these would be really cool to do. that we could learn from and then repeat it at our customers. So that was all with that third dimension that we had in mind.
Robb Boyd: I think that's interesting because this is the same thing that, except for that last one, maybe not as much, but I feel like these are the same dimensions that every customer should be looking at. And as you mentioned, what Jim Kavanaugh was saying as your CEO, I feel like that was something take away the name of the person saying it and this has happened in every company. There's someone in leadership who's saying, Oh my God, this stuff is incredible. I've been playing with it. How can we make this more efficient?
the first thing we mentioned the ATC assistant. So I set you up a little bit talking about. the ATC has been around for, I know World Wide has been around for 30 years, and I think it's been a part of that gathering data, doing proof of concept, documenting what was learned across all these different domains. All that data exists and you guys have that. Is that one of the things that made this attractive to say, how could we work with that data? can you explain that, specifically for us, Dale?
Dale: In the ATC assistant use case as a first, get incepted. We focused on two key data sources, right? The first one being ServiceNow, where it offers really insight and knowledge at the project at client level. And as you said, Robb, WWT has a plethora of experiences across a wide variety of industry and clientele that we can uncover high level at the project level. what are the insights? What are the technologies that we've worked with? What types of companies and industry that WWT has partnered with in the past and what are some high level statistics that we can uncover. Whereas at the more technical detail, Proof of Concept is just one of them that we chose to tackle because of the depth of knowledge and also the technical result that we can extract for business value right there. So those two key data sources are what the ATC assistant first focused on. and Ankur can touch on this maybe in a later section, but essentially, ATC Assistant is something that we created to be merged with the general WWT chat. And, this will create a consolidated view across both WWT platform content, ATC assistant content to form a, unified assistant, if you will, to inform business value for our employees.
Robb Boyd: What would be a workflow that maybe has been in place that you're hoping to affect positively with the chat application? How might that change? Who would be affected? Where do you look to see results? Have you seen anything like that you can share?
Dale: Yeah, absolutely. So I think one use case that we specifically tackle for the ATC assistant is for our engineers to really look into the data in the past to infer upon, new proof of concept that might be tackled in the same technology or the same clientele that we're looking at. For example, if our client is looking at testing SASE technology across two different vendors, and we happen to did a proof of concept in the same row in the past, they can very easily type into the chat, Hey, could you provide me past SASE technology, proof of concept results from so and clients or industry? So that's one way that we use to uncover the value from WWT or ATC Assistant in that matter of fact.
Ankur: The workflow that Dale just described, what we have to keep in mind is that the ATC group of engineers is a very small group that have been around for a long time. And it's like people have all that knowledge in their mind, right? Like we did this with so and so customer like five years back. They have it in their mind that, this was the outcome. And every time like a new request comes in, people have to figure out, Oh, who did that? Whose mind would have that information? So now it's Oh, you don't have to figure out who has that information. All of that information is in a document, which is indexed and ready for you to chat with. So it's like easing the burden on like keeping track of things mentally.
Robb Boyd: That's one of the dreams, is it not? Cause that's institutional knowledge. Cause I used to think about. When I was in sales and we'll talk about why that becomes important in our next project, but, was this notion of, I remember working for companies, and they were careful about getting your laptop back and some different things. And the theoretical thing was, is there's valuable information. That could be used to help the next set of people as the organization, ideally in a perfect world, organizations will get smarter over time. But the problem is a lot of that knowledge is buried in places where it's not that accessible and this is really starting to flesh that out. And with a chat app, we're doing it in human language. So the interface is straightforward, easy to ramp up into. People don't have a huge learning curve. I would assume starting to get productive when these things are built correctly.
Ankur: And that I would also say is just the first interface. The first interface that comes to mind with whenever we talk about GenAI is chat, but we're hoping that these things would penetrate into your, other applications. So for example, whenever you have a Salesforce request that comes in for a lab, then and there you might be able to point them towards like previous labs that we've done that might be of use or similar nature. So instead of the people going over to a separate chat application to talk about stuff. It could be some sort of a integration into an already existing application where it's not even a prompt based thing. It's just a push to you, here's what you actually are thinking about. Here's that information that we already know.
Robb Boyd: The second project is not chat based, I don't believe, but the RFP assistant. Dale, do you mind setting up what is the RFP? What is RFP? I'll have you explain it first, and then I'll jump in with my opinion.
Dale: Sure. So RFP stands for Request for Proposals. And it's oftentimes, RFPs come from vendors or, clients that are looking for a specific solution or services in the area that they'll like to have answers from multiple vendors for, RFP, proposal generation, and also just evaluating whether RFP is worth generating proposal for is a very lengthy process. And that is essentially the use case that we want to tackle. For the RFE assistant, discussed in the paper that you read.
Robb Boyd: Pause there a moment because I just want to share why I think this is so huge. Personal perhaps, but so when I was in sales. RFPs were a mixed blessing because RFPs would come in from some very big customers. Most of them, at least back in my day, were from government side, when I worked on that one. And there's very detailed information. Each one of them is structured differently, so there's no model, there's no standard for how they're going to be written, but yet they all have potentially critically important information, some of which you have to dig through just to find out if you should even respond to the RFP, because it's not automatic that just because someone asked you to bid on a project that you'd want to take it. It may not be a smart business decision, you may not have a compelling advantage over your competition. And so you don't want to play in that. Maybe the margins aren't right, whatever it may be. And so there was this thing that we would always go through and we were on our own. At the beginning, the most we could hope to have from the company I worked for was a overly long, throw everything into a single PowerPoint and then strip out what you don't need to try and fashion it to the RFP you got. And the individual salesperson was responsible for doing that. So a lot of things can go wrong in that process. Not to mention the fact that it'll bog you down so far, you probably miss a lot of business. You guys have a, as I understand from the paper, and this may have changed, but at least 12 people on an RFP proposal team supporting 400 plus salespeople around the world. That sounds like a good ratio, but that's a bottleneck potentially of where they've got to you know, go through a ton of information as quickly as possible to reformat it and say, here's what we think we should do. What kind of things become possible with AI applied to this RFP qualification is the word really, qualification process that becomes so important to business.
Dale: We separate the RFP evaluation also, process there into a couple of stages. So first, before you even evaluate whether the RFP is feasible, we need to understand what it is about. So that is the summarization process. And then we go into the qualification process. Once we have a foothold of understanding of what the RFP is about, we then need to understand, whether it is worth it moving forward. the RFP proposal generation process is often a lengthy one. It often takes our team, from the receival of the RFP to generating the proposal for it to submit it around two, business weeks there. the goal for the RFP assistant is essentially to cut down the processing time. And also the manual labor that our team needs to generate response to these proposals that we receive. And for WWT itself, we can speak to around 500 to 600, RFPs that we receive and process on an annual basis just on the specific team that we're working with. Or there's additional teams such as the government team that also has RFP, RFI that, is outside of the 600 number that I just gave. With that said, when we first went into the RFP assistant, we looked at first, how can we tackle the summarization piece? And also how can we qualify the product? The summarization module, we utilize LLM to give different sections that is predefined by our proposal manager to help our, audience have an understanding of what is the overall asset of the RFP? What is the business value of the RFP? How can WWT response to this RFP? With a competitive advantage, what is the overall timeline and what are some red flags or green flags that we need to watch out for? So the summarization piece itself save our proposal manager a considerable amount of time. Whereas the qualification piece, we pull data from our Salesforce pipeline to essentially quantify how many proposal we have won or lost in this region of the RFP. And what are some historical RFP that you can refer to as a starting point for your response.
Ankur: So this is where we actually embed RFPs, like the, content of the RFP, you embed it and the content of all of the RFPs that you responded to in the past, those are also in that space. So now, this RFP is actually closest to this other file that we did, two years ago, five years ago, one year ago,
Robb Boyd: And you know the results of those,
Ankur: exactly. And then, the results of those, so you have Oh, we've probably got like a hit rate of like X percent with this RFP. So that kind of gives you an intuitive, idea of how much effort to put into this RFP, response. Or even go for it, I don't know not
Robb Boyd: I love how enclosed that process is because it does seem ideal because it's got a very it's got a it's got a start and an end to it that you can define because not everything does and so you can measure how much you've been able to affect things. So with that said, as I set you up, do you have any data you can share at least at a high level of what type of have you seen any change from this? Is there anything you can brag about or say that you've learned perhaps that you would want to do differently or you're working on? Any kind of learnings like that come out of this, that you're. As an example of what you apply back into your client, communication and such too.
Dale: On the summarization piece, typically it takes our proposal manager two to three hours depending on the length of the RFP to go through an RFP, understand it to the point that they can say, hey, we should move on with it or no, we should drop the RFP. with the tool, it takes around three minutes to 10 minutes depending on the length and the document for it to be processed and also for the summarize, sections to be populated. you can do the math, but it went, it goes from two to three hours down to a couple minutes for you to process an RFP. And meanwhile, the time that you have saved is idle time that you can be utilized to do additional tasks at hand.
Robb Boyd: that's what I'm thinking. It feels like in that process, you're more focused on how do we not waste people's time? How do we be more efficient with these tools, which in this case is not the salesperson because he's got an RFP person. So they are sending this over. Is it triggered through Salesforce? What's the high level workflow? For getting that into the process. And then how is it, how does it come back to the customer?
Dale: It is actually a parallel two path. So in the first path and the summarization piece, you very simply, input a RFP itself. The RFP gets summarized by, LMS in the backend to produce the different sections. And these sections are produced by pre engineered prompts that's associated with each section. Whereas on the qualification piece, it is another parallel path that utilizes Salesforce as the back end data sources. The exact process is very complicated. So essentially, I'll give you a summarized version of it. The RFP that you input into the solution is summarized with a description, whereas all of the RFP's or past RFP's in Salesforce is also summarized into their own, data information that's stored in the VectorDB. In the backend, we utilize LLM to actually assign cosine similarity that places, similar RFPs closer to the RFP that you submitted to give you a stacked range, similar RFPs in the past that you can take a look at as well.
Robb Boyd: So could you literally come out of this and say, if, assuming someone can't reply to all of them, you can rank them based on value of some sort as well to know what is right for the business. You're actually tweaking maybe in more dimensions than you could have.
Ankur: This is just one part, right? Like the summarization and the qualification, which would normally span like one to three business days is now down to a few minutes or maybe hours, but then the, main meat is also on the proposal writing. So how do you actually respond? And that's a development we've had since the paper was written. So that's maybe not something you already know of Robb, but as of like yesterday or last week, We have the tool in beta that the proposals team is testing on where they also get like a first response of the draft and that those responses are based off of historical RFPs. Yes. But also on, the platform data, the content on wbt. com external as well as internal that's available to our, account managers and sales force, as well as, a bunch of other, proprietary data sources, including Salesforce and whatnot. All of those together are used to do, get a first draft of the response in front of the, proposal manager. And they were like, Oh, this is 70 percent there maybe, and this takes, a bunch of time away from like the, time it took for us to create a first draft and put it in front of a SME so that they could validate what we've written is right. Or, suggest changes. All of that is now pretty fast. We can just take this first response, put it in front of an SME and then work on top of it.
Robb Boyd: And I would think that as you guys, the number of submissions that you got for ideas for what to approach a year ago when you started on these, or whatever it was, I'm guessing at the numbers, but I would imagine as we get smarter as individuals and we're looking at our processes different now, there's got to be more people banging on your door. I would imagine internally going, wait, I want to do this with service contracts or, something else to help us optimize. You guys may have started on that. That's not the point. What I love is the twist is here. You guys are an enterprise that is doing what you need for your business. And you're using that as an educational basis. As you mentioned, learning being that other dimension that becomes very important because that's what every customer is going through, which is, this is what we want to do in our operation. How do we do it intelligently? Have you learned anything you could highlight that you've learned that you would have done differently or that you, or you're glad you did, or do you have to go back and redo some things? Any kind of learnings like that come out of this, that you're. As an example of what you apply back into your client, communication and such too.
Dale: When we first talk with the stakeholders, even within our company itself, a lot of times we focus on the value and complexity piece. But we typically often overlook the part, the, where, data plays a role in terms of, feeding into the LLM pipeline. And this is something that we learn throughout the way, as we uncover use cases that we want to do. But the data and the data governance is not in place in a way that will actually allow us to extract Insight, and feed it into the LLM layer itself. So I think that is a lesson that we learned, through a hard way, right? Some of the use cases we tried to tackle, but the data is simply not available, or not in a format that is conducive to actually getting utilized by LLM itself. So I think that's one lesson that we learned that, look, moving forward. In the selection of use case, looking at the data and data availability is a key thing that I think every organization should do.
Ankur: So Dale talked about selection of the use case. On the technical side, I would say selection of the technology. like a year ago, all of this is brand new, right? So a year ago, there were some names in our minds, right? Which were like, Oh, this has to be the best option. Let's just go with that. And without naming specifics, we were like, Oh, this is like a well established player in this certain space in the vector DB technology or whatever. And now that I'm looking back, like eight months, nine months into this development, we're not using any of those, names that we had in mind to go start off with, it's, basically a bunch of new companies that did not even exist, maybe an year ago, or maybe they existed, but they were pretty small that have taken up this opportunity and scaled up their products or, given us something differentiator that's actually being used. and a lot of those are in the, orchestration and the exchange layer, open source things like Lang chain or Namas with, and all of those things, which did not exist a year ago. But also on the proprietary side of of vector DB's like Pinecone or whatever, without naming too many specifics.
Robb Boyd: Yeah, but here's what, and check me if I'm understanding this correctly, but to circle back around, this is the power of the framework that you guys are following. Yeah. In that these things are going to change again a year from now. There's a bunch of names we haven't heard of. There could be some surprising changes. I'll call out the one thing that we're all probably aware of in the tech industry that happened, but it's like, who knew what was going to happen with Open AI and then how they went from the model that they've been at all the discussions about, what's Microsoft's involvement? And how does that mean? what does that mean for where it's going in the future? And so many big questions. Either way, we're working with decision makers and our audience who have to make decisions about what do I do today to be able to accelerate it. my ability to manipulate in this market, to win in this market, because everything is moving fast, but yet we can't ignore it, but we can't jump too fast. So that is why I really like what you guys are doing. I want to just spend the last few minutes and have you comment on how do you suggest customers engage with you guys in this area?
Ankur: Majority of the times where we, come in is usually when, VPs or execs of certain departments or business areas are looking at a holistic approach towards AI. They're like, I want all of this operations or all of this decision making to be driven through data and driven through AI. That is where we come in through a transformational approach, and we're like, part consultants, part data scientists, and part, you hardware experts, that's what WWT is. The backing of a hardware company which can, talk about the engineering side of things. We've got our best talent in terms of consulting who can understand business operations and business challenges. And we've got data scientists and data engineers who can work with the data and provide the solutions on top of this. So whenever there's like a transformational change or a paradigm shift that companies are looking at, that's essentially our top differentiator where we can come in and do end to end, not just consult and go away, but consult. recommend the hardware, buy the hardware, install the hardware, work with the data, deliver outcomes, and then go back.
Dale: Depending on your maturity and what you're tackling in terms of GenAI use case, we can help you in any way, right? Whether you're looking to evaluate different LLM models or looking at the different hardwares that you should support from IT infrastructure perspective, those are all at hand either through ATC or also through our consultant services that we are available to help. And from our experiences building our own internal use case, I think we are very well positioned to advise you either you're looking at a very specific use case or you have no idea where to start.
Robb Boyd: Anker Gupta, thank you so much for your time.
Ankur: Thank you, Robb great to be talking to you as always.
Robb Boyd: Absolutely. And Dale, thank you for your time as well.
Dale: Thank you so much, Robb for hosting us.
Robb Boyd: I'm reminded of a quote. "By far, the greatest danger of artificial intelligence is that people conclude too early that they understand it." And that's where y'all come in. WWT has loads of resources to help you make top notch choices and investments in AI. At a minimum, I encourage you to check out today's featured research paper, "How WWT is Harnessing Generative AI to Drive Internal Business Value." You're going to find that link and more below. the best time to jump on this was yesterday. That makes today the next best thing. My name is Robb Boyd. Thank you for watching.